What Can Digital Patient Twins Do For Clinical Research?
By Rene-Pascal Fischer, scientist & software architect, Fraunhofer IESE
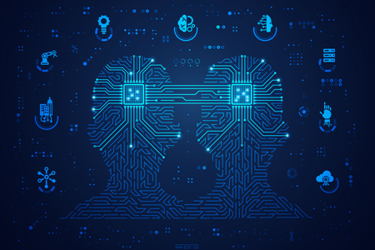
Originating from the realms of Industry 4.0 and Pharma 4.0, digital twins have traditionally been associated with optimizing manufacturing processes and facilitating the advent of individualized medicine, represented by the concept of "lot size one" production. However, their transformative capabilities extend beyond the boundaries of industrial settings to the very core of healthcare, offering many prospects for personalized patient care.
At the core of digital twins lie physical and logical assets that benefit from digitalization. This paradigm holds true for patients as well. Just as digital twins revolutionize the understanding and optimization of machinery and processes, they offer a unique opportunity to create virtual models of individual patients, capturing their physiological nuances and health attributes with high accuracy.
We call them digital patient twins (DPTs). These DPTs serve as virtual replicas of patients, crafted from population data, individual health records, and real-time feedback from wearable devices. Each DPT encapsulates a comprehensive snapshot of an individual's health journey, ranging from genetic predispositions to daily activity patterns captured by wearable sensors, going as far as using simulation technology for predictions on individual health responses.
While the concept of DPTs holds immense promise for revolutionizing healthcare, it's important to acknowledge that a fully functional DPT remains a visionary goal. However, this vision is steadily taking shape, propelled by advancements in data science, wearable technologies, and AI. Researchers and innovators are diligently working to refine methodologies for DPT creation, integrating diverse streams of health data and enhancing predictive capabilities.
The Current DPT Landscape
While facing challenges in comprehensive model development and reliable drug response predictions, DPTs show promise in transforming healthcare by integrating data from wearables and other health-related sources, even if we still haven’t witnessed implementations of the concept. 1-3 Initiatives like the Swedish Digital Twin Consortium underscore the potential of DPTs in personalized medication and the need to take the path toward digitalization.4 Furthermore, DPTs hold promise in guiding the design of individualized therapeutics and facilitating automated pharmaceutical manufacturing processes.5
Regulatory hurdles, scalability issues, and the need for enhanced digitalization in healthcare remain key obstacles, emphasizing the importance of ongoing research and development efforts.6, 7 Nevertheless, DPTs hold significant potential for precision medicine, bridging gaps between health monitoring, pharmaceutical manufacturing, and regulatory requirements.8 As access to individual health information grows, DPTs could play a pivotal role in reshaping healthcare systems, provided regulatory aspects and quality control systems are effectively addressed.
The Potential Of DPTs In Clinical Trials
One of the most compelling aspects of digital twins, in general, lies in their ability to simulate assets with remarkable accuracy.9, 10 This could be extended to the realm of clinical trials. Currently, clinical trials face significant challenges in predicting how patients will react to various medications, at times leading to unforeseen adverse effects and suboptimal treatment outcomes. Additionally, clinical trials are difficult to design and expensive to operate, making digitalization not only useful for patient safety but also the economics of drug development. DPTs offer a potential solution to this problem by providing a platform for conducting simulations prior to in-human testing. Building simulation models based on a patient's genetic information and health data, DPTs could be used to predict potential side effects and tailor treatments to maximize efficacy while minimizing safety and financial risks.11, 12
Furthermore, DPTs offer a pathway to more accessible and inclusive clinical trials. Historically, clinical trials have struggled to recruit diverse patient populations, leading to gaps in our understanding of how different demographic groups respond to treatments. However, DPTs have the potential to overcome these barriers by providing a virtual platform for conducting trials in silico. By simulating the responses of diverse patient populations, researchers can gain insights into how various demographic factors influence treatment outcomes, ultimately leading to more inclusive and representative clinical trials.
This aspect also introduces the possibility of enhancing clinical trials with larger sample sizes, as scaling can be done completely virtually. In combination with concepts for continuous engineering, small changes in drugs that may improve cost efficiency or general availability could be calculated during the early stages of drug development, making drugs not only safer but also cheaper for the public. Additionally, we could leverage these ideas to improve individualized therapies even further, as patient-specific drugs may not have enough economic incentives in the current drug manufacturing area. With digitalized technologies, and potentially clinical trials, even small batches can be economically viable.
In conclusion, the possibilities of DPTs have a high potential to enhance patient safety, introduce more individualized therapies, and promote inclusivity in medical research. By harnessing the power of data science and AI, DPTs can transform the way we approach healthcare, ushering in a new era of personalized medicine and precision therapeutics.
Data Interoperability And Standardization Among Top Challenges
Achieving a future where DPTs revolutionize clinical trials and healthcare requires concerted effort and investment across multiple fronts. First, we must continue to pursue advancements in data science and AI, enabling the creation of more sophisticated and accurate DPTs. This involves refining algorithms for data analysis, improving predictive modeling capabilities, and enhancing the integration of diverse data sources.
Additionally, collaboration between healthcare providers, pharmaceutical companies, technology developers, researchers, and regulatory agencies is essential. This collaborative approach ensures that DPTs are developed following rigorous standards for safety, efficacy, and data privacy. Furthermore, ongoing research is needed to validate the accuracy and reliability of DPTs in real-world clinical settings and demonstrate their value in improving patient outcomes and reducing healthcare costs.13
Moreover, efforts to enhance health data interoperability and standardization are crucial for maximizing the utility of DPTs across different healthcare systems and settings. This involves developing common data formats, protocols, and infrastructure for sharing and exchanging health information seamlessly.
Collaboration Needed To Bring DPTs To Fruition
The potential of DPTs to improve our current healthcare systems is immense, offering personalized therapeutics, enhanced health monitoring, and streamlined pharmaceutical manufacturing. While the journey to realizing this vision may seem daunting, it's important to remember that change is within our reach; achieving the vision of DPTs transforming clinical trials and healthcare is a collective endeavor. Regulatory agencies, such as the FDA and EMA, have started their own programs 14, 15 helping to incorporate new ideas such as simulation into the development of new drugs.16-18 By working together, stakeholders can harness the full potential of this technology to drive innovation, improve patient care, and advance the healthcare sector as a whole.
By fostering collaboration, investing in research and development, and prioritizing innovation, we can collectively shape a future where DPTs play a central role in optimizing patient care and driving advancements in healthcare. Each of us, whether as healthcare professionals, researchers, policy makers, or advocates, has the power to contribute to this transformative journey. By simply starting the conversations we will get one step closer to achieving the goal of DPTs. Together, we can harness the capabilities of DPTs to improve outcomes, increase accessibility, and ultimately, change the landscape of healthcare for the better.
References:
- Smuck M, Odonkor CA, Wilt JK, Schmidt N, Swiernik MA. The emerging clinical role of wearables: factors for successful implementation in healthcare. NPJ Digit Med. (2021) 4(1):45. doi: 10.1038/s41746-021-00418-3
- Chandrasekaran R, Katthula V, Moustakas E. Patterns of use and key predictors for the use of wearable health care devices by US adults: insights from a national survey. J Med Internet Res. (2020) 22(10):e22443. doi: 10.2196/22443
- Dunn J, Runge R, Snyder M. Wearables and the medical revolution. Per Med. (2018) 15(5):429–48. doi: 10.2217/pme-2018-0044
- Björnsson B, Borrebaeck C, Elander N, Gasslander T, Gawel DR, Gustafsson M, et al. Digital twins to personalize medicine. Genome Med. (2020) 12:1–4. doi: 10.1186/s13073-019-0701-3
- Sun T, He X, Li Z. Digital twin in healthcare: recent updates and challenges. Digit Health. (2023) 9:20552076221149651. doi: 10.1177/20552076221149651
- Colloud S, Metcalfe T, Askin S, Belachew S, Ammann J, Bos E, et al. Evolving regulatory perspectives on digital health technologies for medicinal product development. npj Digit Med. (2023) 6(1):56. doi: 10.1038/s41746-023-00790-2
- European Federation of Pharmaceutical Industries and Associations. EFPIA Reflection Paper on the Need for Better Defined Regulatory Pathways in the EU for Digital Health Technologies used concomitantly with Medicinal Products or as drug development tools during Clinical Development. (2021). Available online at: https://www.efpia.eu/media/636454/efpia-rp-digital-health-technologies_november-2021.pdf
- Swen JJ, van der Wouden CH, Manson LE, Abdullah-Koolmees H, Blagec K, Blagus T, et al. A 12-gene pharmacogenetic panel to prevent adverse drug reactions: an open-label, multicentre, controlled, cluster-randomised crossover implementation study. Lancet. (2023) 401(10374):347–56. doi: 10.1016/S0140-6736(22)01841-4
- Grieves M, Vickers J. Digital twin: mitigating unpredictable, undesirable emergent behavior in complex systems. In: Kahlen J, Flumerfelt S, Alves A, editors. Transdisciplinary Perspectives on Complex Systems: New Findings and Approaches. Cham: Springer International Publishing (2017) p. 85–113. doi: 10.1007/978-3-319-38756-7_4
- Zheng Y, Yang S, Cheng H. An application framework of digital twin and its case study. J Ambient Intell Humaniz Comput. (2019) 10:1141–53. doi: 10.1007/s12652-018-0911-3
- Grimstein M, Yang Y, Zhang X, Grillo J, Huang SM, Zineh I, et al. Physiologically based pharmacokinetic modeling in regulatory science: an update from the US food and drug administration’s office of clinical pharmacology. J Pharm Sci. (2019) 108(1):21–5. doi: 10.1016/j.xphs.2018.10.033
- Nijsen MJ, Wu F, Bansal L, Bradshaw-Pierce E, Chan JR, Liederer BM, et al. Preclinical QSP modeling in the pharmaceutical industry: an IQ consortium survey examining the current landscape. CPT Pharmacometrics Syst Pharmacol. (2018) 7(3):135–46. doi: 10.1002/psp4.12282
- Fischer R-P, Volpert A, Antonino P and Ahrens TD (2024) Digital patient twins for personalized therapeutics and pharmaceutical manufacturing. Front. Digit. Health 5:1302338. doi: 10.3389/fdgth.2023.1302338
- U.S. Food and Drug Administration. Guidance for industry: physiologically based pharmacokinetic analyses — format and content. (2018). Available online at: https://www.fda.gov/media/101469/download
- European Medicines Agency. Guideline on the reporting of physiologically based pharmacokinetic (PBPK) modelling and simulation. Reference No. EMA/CHMP/458101/2016. (2018). Available online at: https://www.ema.europa.eu/en/documents/scientific-guideline/guideline-reporting-physiologically-based-pharmacokinetic-pbpk-modelling-simulation_en.pdf
- Colloud S, Metcalfe T, Askin S, Belachew S, Ammann J, Bos E, et al. Evolving regulatory perspectives on digital health technologies for medicinal product development. npj Digit Med. (2023) 6(1):56. doi: 10.1038/s41746-023-00790-2
- U.S. Food and Drug Administration. Framework for the use of digital health technologies in drug and biological product development. (2023). Available online at: https://www.fda.gov/media/166396/download?attachment
- European Medicines Agency. Mandate of the EMA Innovation Task Force (ITF). Reference No. EMA/484400/2014. (2014). Available online at: https://www.ema.europa.eu/en/documents/other/mandate-european-medicines-agency-innovation-task-force-itf_en.pdf
About The Author:
Rene-Pascal Fischer is a scientist and software architect at Fraunhofer IESE, focusing on the topic of Industry 4.0 and its adoption in the pharmaceutical domain. Having started his Ph.D. on the topic of validation and certification of processes using digital twins, he is an advocate of holistic digitalization and the connected enablement of a digital control flow. He is actively contributing to open-source software and multiple research projects for both industry and Pharma 4.0 as well as the CoP Pharma 4.0 of the ISPE. Fischer can be reached via email or on LinkedIn.