4 Perspectives On What's Next In Clinical Trial Tech
As told to Abby Proch, executive editor of guest columns, Clinical Leader
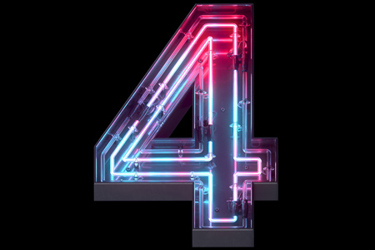
It’s that time of year again — time to reflect on the past 365 days and look ahead to the next ones. In this 2024 wrap-up, we’re talking about clinical trial technologies. Surprisingly, when asked, folks didn’t offer much criticism of existing technologies. Rather, they took a rosier look at the promises future tech might hold.
Clinical Leader asked them: “Internal, external, patient-facing, or otherwise — what clinical trial tech tool are you most excited to embrace or ditch in the new year? And why?”
Here’s what four experts across the industry had to say.
“I expect AI to hit the top five. While AI implementation in medicinal product development has been growing exponentially, clinical trials are yet to unlock the full AI potential to become more effective. Currently, AI is mostly used to identify potential study sites, in medical writing, and in risk-based monitoring. It can do much more, e.g., support risk-benefit assessments, evaluate the impact of protocol amendments on study timelines and budgets, help monitor participants’ safety, identify risks to data quality before they become issues, etc. Adoption requires prudence. Any AI tool performs as it has been trained, hence, the quality of training data sets must be ensured. This includes data provenance, privacy and security considerations, testing, validation, human oversight and decision-making.
I am curious to see what 2025 brings. And what I would like to see sinking in history? Endless spreadsheets and petrified templates that are not fit for purpose anymore.” –Kamila Novak, principal, KAN Consulting MON. I.K.E.
“Earlier this year, DiMe worked with academic and industry colleagues to quantify the financial return on investment associated with the use of digital endpoints. I’m excited to see how trial sponsors leverage these findings to transform the net present value calculation for development programs deemed ‘not commercially viable.’
“Our findings show that digital endpoints can reduce the number of subjects needed for trials and reduce overall trial timelines, which will be a huge boon for studies where enrollment is particularly challenging. Take rare diseases, for example. There are very few patients to begin with, and while advances in genomics and precision medicine have created immense promise for these patients, the lack of clinical and commercial viability of programs in this space often halts progress in its tracks.
“I think digital endpoints can change this by accelerating the development and commercialization of disease-modifying therapies. I’m looking forward to seeing where we go from here!” –Sarah Valentine, partnerships lead, DiMe
“In my work as a clinical development expert, I’m most excited about the potential of clinical trial tech tools that can calculate a patient’s placebo-covariate. Tools like Placebell from Cognivia reduce the noise caused by the placebo response, which results in decreased data variability and improved statistical analysis.
“In diabetes trials, demonstrating efficacy is primarily driven by the decrease in HbA1c levels. However, the significant variability of this measure poses a substantial challenge in proving efficacy, as this variability complicates the comparison between treatment groups. Prognostic covariates that combine machine learning with patient psychology allow researchers to identify differences in cultural backgrounds, personal beliefs about health and medicine, and socioeconomic factors. This information allows us to adjust trial design accordingly, ensuring treatments are evaluated fairly across diverse populations. Technology like this is essential to promoting DEI initiatives, reducing the failure rate, and supporting innovation.” –Jean Van Rampelbergh, chief clinical development officer, Imcyse
“The integration of AI in clinical trials extends beyond target discovery, ushering in a paradigm shift essential to advancing clinical trials in 2025 and beyond. A biology-first approach to AI plays a pivotal role in addressing challenges and unlocking efficiencies in clinical trials. Unlike generic applications, causal AI models designed to assimilate data from patient biology can provide insights that guide trial design, patient selection, and safety monitoring. By leveraging AI to understand the biological mechanisms behind drug actions, researchers can identify which patients are most likely to respond favorably and tolerate treatment, thereby improving trial outcomes.
“AI-driven analyses can also anticipate potential adverse events and suggest protocol modifications, such as incorporating complementary treatments to mitigate side effects. This informed approach supports the safety and efficacy of trials and can accelerate the path to approval, ultimately benefiting a wider patient population.” –Niven Narain, Ph.D., president and CEO, BPGbio