Clinical Data Needs Smart Automation Now for a Better AI Future
By Edward Jones, Senior Director, Strategy, Veeva CDB
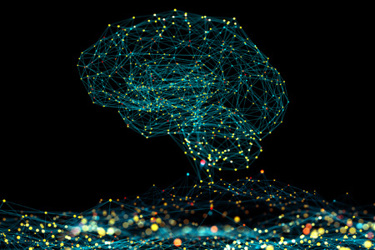
Artificial intelligence (AI) and machine learning (ML) have shown great promise in drug discovery, aiding in target identification, molecular simulations, and drug property predictions. These technologies handle vast amounts of molecular data, enabling them to detect patterns and make significant discoveries. Companies like Novo Nordisk have leveraged AI to enhance quality control and commercialization. However, the application of AI and ML in clinical study execution is still in its early stages.
Unlike drug discovery, clinical data from trials often does not qualify as "big data," making it difficult to train complex ML models. While AI is not yet a proven solution for improving Clinical Data Management (CDM) processes, recent advancements in automation have significantly reduced cycle times and costs. Automation has also improved data quality, yet the industry remains distracted by the AI hype, underutilizing these practical automation solutions. Ethical considerations and regulatory compliance further complicate AI/ML adoption in CDM.
As the industry continues to evolve, there is a pressing need to balance the immediate benefits of automation with the potential long-term advantages of AI, ensuring clean data is a fundamental requirement.
Get unlimited access to:
Enter your credentials below to log in. Not yet a member of Clinical Tech Leader? Subscribe today.